The Intersection of Large Language Models and Human Expertise
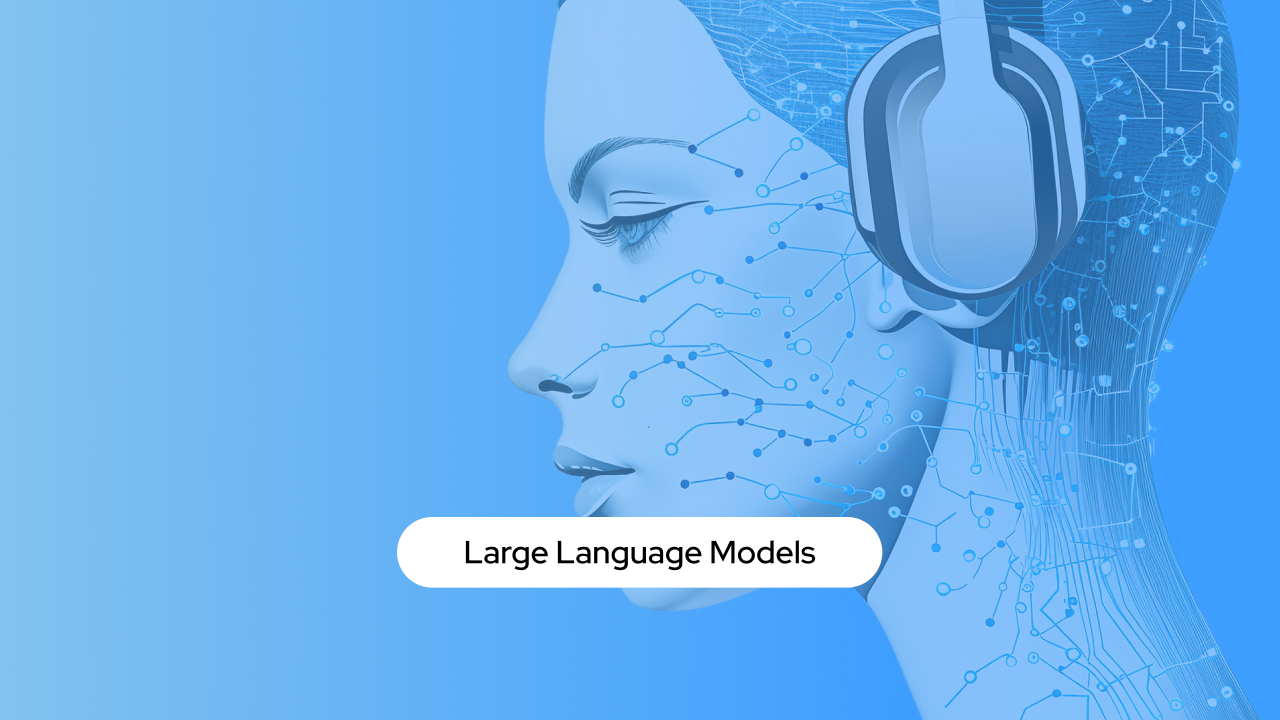
Artificial Intelligence (AI) is not a solo act. It thrives at the intersection of advanced algorithms and human intelligence, especially in the context of Large Language Models (LLMs) such as GPT (Generative Pretrained Transformer). LLMs have brought about a revolution in AI, largely due to their prowess in classification—the process of sorting data into predefined classes. This capability is becoming the backbone of a new era of AI applications, impacting everything from customer service to personalized marketing.

The Pivotal Role of Classification in AI
At its core, classification is a type of supervised learning that teaches a model to interpret inputs and organize them into relevant categories. This is particularly crucial in managing the deluge of unstructured data our digital world generates. Through classification, businesses are not just interpreting this data; they're leveraging it to drive decisions, enhance user experiences, and streamline operations.
How LLMs Excel in Classification
LLMs interpret and classify data by utilizing neural networks trained across extensive text corpora. These networks generate vector spaces that convert words, phrases, and documents into mathematical entities. By doing so, they capture the subtleties of language, allowing for nuanced groupings based on contextual relationships, not just literal ones.
What sets modern LLMs apart is their proficiency in 'few-shot' or 'zero-shot' learning. They can tackle new classification tasks with little to no additional training. This rapid deployment capability ensures LLMs can be versatile tools in diverse settings, providing contextual understanding and content categorization with ease.
Practical Benefits of LLMs in the Real World
Classification via LLMs serves as a beacon of efficiency in customer service, swiftly sorting through and responding to customer queries. In the realm of content moderation, these models ensure digital environments adhere to community standards. And in marketing, LLMs harness classification to deliver targeted, personalized content that resonates with individual preferences, thus boosting engagement and satisfaction.
Enhancing LLMs: The Strategic Role of Staff Augmentation and Data Annotation
However, the true efficacy of classification in LLMs is not solely a product of their design. It's also dependent on the quality of data and the expertise involved in preparing it. This is where the strategic roles of staff augmentation and data annotation come to the fore.
Bolstering AI with Staff Augmentation
Staff augmentation allows AI companies to enhance their workforce with temporary, specialized staff who can support the intricate needs of LLMs, especially in the subtle art of classification. These augmented teams are crucial for tasks like data curation, model optimization, and iterative improvement, ensuring that the LLMs operate at their peak.
Data Annotation: The Backbone of Accurate Classification
Data annotation involves tagging data so that LLMs can understand and learn from it effectively. Properly labeled data sets are essential for training LLMs to classify inputs accurately. The meticulous effort put into data annotation influences the model's ability to discern and categorize correctly.
Symbiosis Between Human Expertise and Data Annotation
A symbiotic relationship emerges between staff augmentation and data annotation, with each aspect reinforcing the other. Augmented experts optimize the annotation process, and in turn, well-annotated data enables the training of more accurate and reliable LLMs.
Setting the Path for Future AI
As LLMs continue to evolve, their classification abilities will only become more sophisticated, promising a future where AI's categorization and response mechanisms are indistinguishable from human capabilities. The joint forces of staff augmentation and data annotation are not just operational necessities but strategic investments that fuel this evolution. They empower AI systems to be not just intelligent but also equitable and unbiased.
For AI companies, recognizing and investing in the synergy between LLMs, staff augmentation, and data annotation is not just about gaining a competitive edge. It's about paving the way for transformative AI, where the convergence of human expertise and machine intelligence sets new standards for efficiency, innovation, and engagement in the information age.