Why AI can't draw human hands? The role of image annotation and ML models training in generative AI

Why Do AI Models Struggle with Drawing Human Hands Accurately?
The answer is straightforward – insufficient training.
In generative AI, the process of data annotation and labeling is essential. These elements are indispensable for the existence of the first.
Imagine AI as a visitor confined in a museum, where learning is limited to what is visible within its walls. This situation underscores the critical need for not just a large quantity of data but also its quality, especially for generative models to yield satisfactory outputs.
Learn more about the intricacies of data annotation in generative AI in our most recent article:
Why can’t AI draw human hands? The role of image annotation and ML models training in generative AI
If you request a generative AI program like DALL-E or Midjourney to illustrate a human hand, the results are now quite impressive. However, this level of accuracy wasn't always achievable. Previously, these generative models struggled to produce anything remotely resembling a hand. This shortcoming was primarily due to a lack of sufficient input data for learning. The databases simply didn't contain enough varied examples of human hands.

Despite improvements, rendering human hands remains a challenge for generative AI. This difficulty arises from the intricate biomechanics of hands, aspects that are not easily discerned from static images. While AI has become adept at understanding how objects appear, grasping their functional mechanics is another story.
The learning process of AI is confined to the data it has been exposed to. Unlike humans, who constantly encounter a rich array of visual examples in the real world, AI's learning is limited to its pre-existing data pool.
It's not just the volume of data that matters, but also its quality. This is where data annotation becomes crucial. Properly labeled and annotated data are essential for training not only generative models but all kinds of AI and machine learning systems.
Another specific challenge with hands is their movement, which is more diverse compared to facial expressions, for instance.
Why can't AI simply retrain on more data? The answer lies in the immense computational power required - for instance, operating ChatGPT alone consumes about 564 MWh of electricity daily. In contrast, data annotation is a more resource-efficient approach, relying on human input rather than computational resources. The upvote and downvote feedback mechanism in recent ChatGPT updates exemplifies data annotation. Users indirectly train the AI by indicating their preferences, guiding it to generate more desirable responses.
Data annotation and labeling are critical components in the field of AI. Recent studies indicate that by 2030, the market for these services is expected to surge to an impressive $17.1 billion.
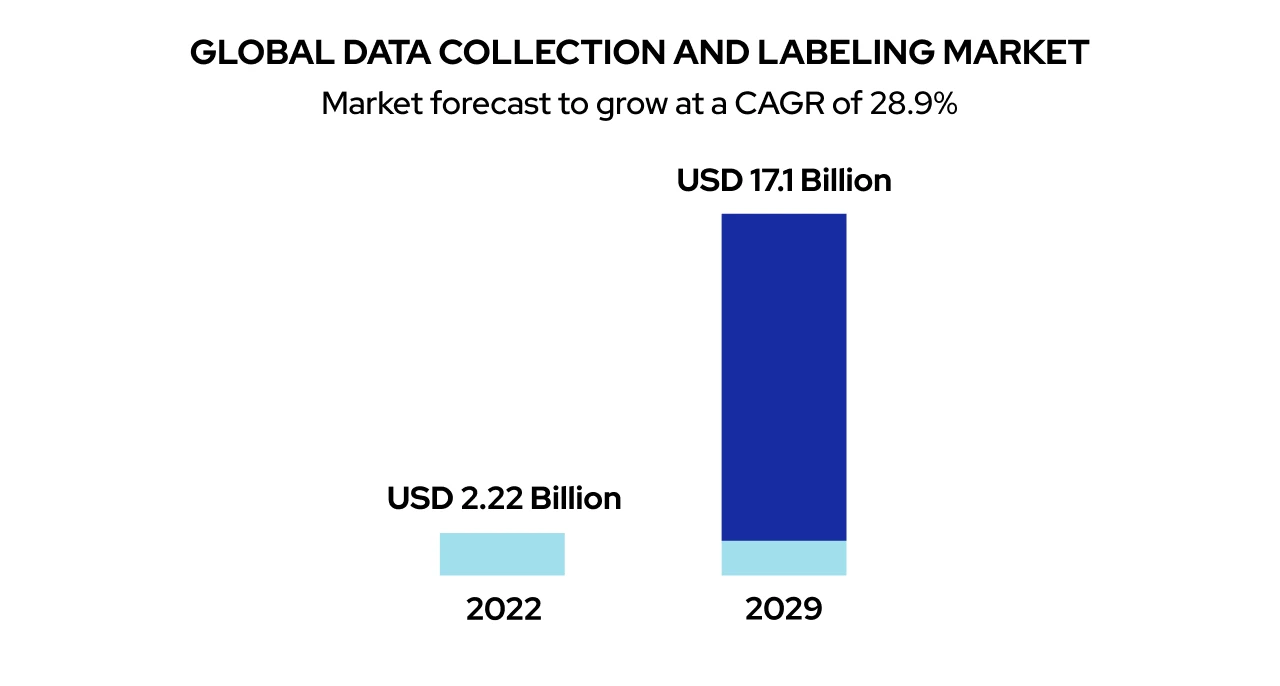
Regardless of an algorithm's sophistication, it's important to remember that it is developed by humans and requires our guidance for effective training. This training is essential for ensuring that we get appropriate responses from AI systems. AI LABS specializes in providing professional data annotation services, supporting AI companies in their development and growth. Stay updated with us for the latest insights into the AI industry.